Unlock the Secrets: How AI Voice Recording Transforms Sound into Text Magic!
In today's fast-paced digital world, the ability to convert audio to text has become increasingly important. AI voice recording technology has emerged as a powerful tool that streamlines this process, allowing individuals and organizations to capture spoken words with remarkable accuracy. From business meetings to educational lectures and media productions, this technology plays a crucial role in enhancing productivity and accessibility. As we delve into the fascinating world of AI voice recording, we will explore the underlying technologies, the conversion process, and the myriad applications that are reshaping how we communicate and share information.
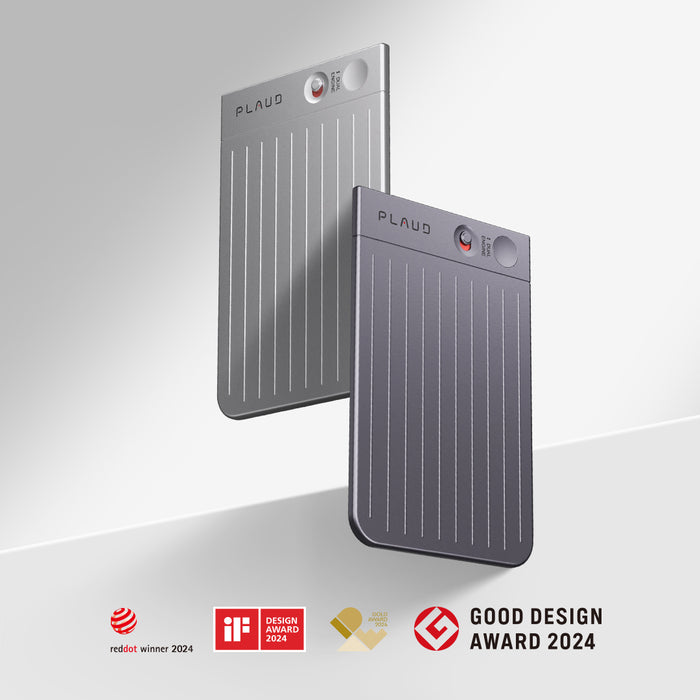
Understanding AI Voice Recording Technology
The backbone of AI voice recording lies in advanced technologies such as natural language processing (NLP) and machine learning. NLP enables machines to understand and interpret human language, breaking down the complexities of spoken words into manageable data. Machine learning, on the other hand, involves training algorithms with vast amounts of audio data so they can recognize patterns and improve over time. These technologies work in harmony: NLP processes the audio input, while machine learning continuously refines the system's accuracy based on user interactions and corrections. The result is a sophisticated voice recognition system capable of converting audio into text with increasing precision, making it an invaluable asset across various sectors.
The Process of Converting Audio to Text
The journey from sound to text involves several key steps. First, audio capture occurs through microphones or recording devices, which digitally encode the sound waves. Once captured, the audio is processed through a speech recognition engine that breaks it down into phonemes—small units of sound. The engine then analyzes these phonemes using algorithms informed by machine learning models. These models compare the sounds to a vast database of known words and phrases, allowing the system to generate a text output that reflects the original audio. Factors such as the clarity of the speaker's voice, the presence of background noise, and the complexity of the language all influence this process. Continuous advancements in algorithm development and the incorporation of user feedback are essential for refining accuracy and ensuring that the technology adapts to various speaking styles and accents.
Applications of AI Voice Recording in Various Fields
AI voice recording technology is making significant strides across multiple industries. In healthcare, for instance, doctors use voice-to-text software to quickly document patient interactions, enhancing efficiency and reducing administrative workloads. The legal field benefits from transcripts of court proceedings, allowing lawyers to focus more on their cases rather than note-taking. In education, teachers can record lectures and provide students with transcripts, catering to different learning styles and ensuring all students have access to the same material. The media industry has also embraced this technology, utilizing it for transcribing interviews and creating captions for videos. These applications not only improve productivity but also foster more inclusive environments by making information accessible to a broader audience.
Challenges and Limitations of AI Voice Recording
Despite its numerous advantages, AI voice recording technology is not without challenges. One significant hurdle is the variation in accents and dialects, which can lead to misinterpretation of words. Additionally, background noise can interfere with audio clarity, complicating the recognition process. Context understanding remains another limitation; while AI has come a long way in recognizing specific phrases, it can still struggle with nuances and idiomatic expressions. Researchers are actively working to overcome these issues by improving algorithms and training models with diverse datasets that include various accents and speech patterns. As advancements continue, the goal is to create a more adaptable system that can handle the complexities of human language.
Embracing the Future of AI Voice Recording
The transformative impact of AI voice recording on modern communication is undeniable. As technology continues to evolve, the potential for converting audio to text efficiently and accurately will only grow. Embracing this technology can lead to significant improvements in productivity and accessibility across various fields. As we look to the future, it is essential to consider the implications of AI voice recording and explore how it can be harnessed to enhance our everyday lives and professional environments. Whether you're a business professional, educator, or content creator, the magic of AI voice recording is poised to revolutionize how we communicate.